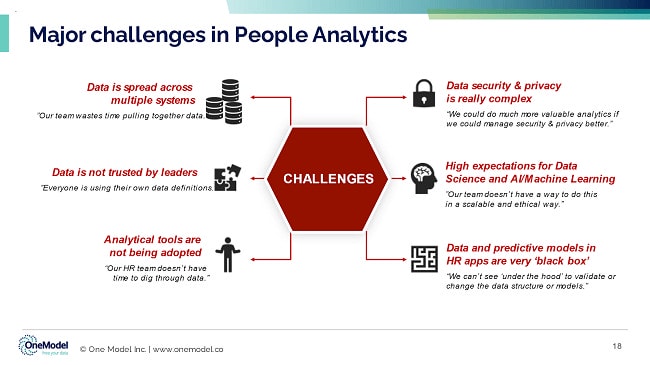
What Are the Challenges of Data Analytics? Let’s dive into this exciting topic! Data analytics is all about uncovering valuable insights from vast amounts of data. But, like any journey, there are challenges along the way. In this article, we’ll explore the obstacles that data analysts face and how they overcome them.
Data analytics is like solving a mind-bending puzzle. It requires a mix of technical skills, critical thinking, and creativity.
But imagine trying to solve that puzzle when the pieces keep changing shape and color! That’s one of the challenges of data analytics: dealing with the sheer volume and variety of data. It’s like searching for a needle in a haystack, and the haystack keeps growing.
Another hurdle is ensuring the quality of the data. Picturing your data as superheroes, you want your Avengers, not a bunch of random strangers, right? Poor data quality can lead to inaccurate results and flawed insights. So, data analysts need to scrub, clean, and validate their data to make sure it’s reliable and trustworthy.
In summary, the challenges of data analytics are like navigating through a maze filled with ever-changing shapes and sizes. It’s a thrilling adventure for those who love exploring the hidden treasures within data. So, put on your detective hat, sharpen your analytical skills, and let’s uncover the secrets that data holds!
What Are The Challenges Of Data Analytics
When it comes to data analytics, there are several challenges that organizations face. One of the main challenges is dealing with large volumes of data and extracting meaningful insights from it. Another challenge is ensuring data quality and accuracy, as inaccurate data can lead to faulty analysis.
Additionally, data privacy and security are major concerns in the field of data analytics. Finally, there is also the challenge of finding skilled data analysts who can effectively analyze and interpret data. Overcoming these challenges requires robust strategies and advanced tools.
1. Data Quality and Integrity
Data quality is perhaps the most fundamental challenge in data analytics. The success of any analysis relies on the accuracy, completeness, and consistency of the data being examined. Inaccurate or incomplete data can lead to misleading conclusions and ineffective decision-making.
Additionally, maintaining data integrity becomes even more complex when dealing with large volumes of data from diverse sources. Data cleaning and preprocessing techniques are critical to ensure the reliability of the insights derived from data analytics, but they require significant time and effort.
Ensuring Data Quality
To address data quality challenges, organizations should establish rigorous data governance practices. This involves defining data standards, implementing data validation processes, and regularly monitoring data quality metrics.
It is also crucial to establish clear protocols for data collection and storage to minimize the chances of errors or inconsistencies. Conducting regular data audits and investing in advanced data cleansing tools can further enhance data quality and integrity.
2. Lack of Skilled Talent
The field of data analytics requires a unique combination of technical expertise and business acumen. Finding professionals who possess the necessary skills to navigate and extract insights from complex datasets can be a significant challenge for organizations.
Additionally, there is a shortage of data scientists and analytics professionals in the market, making it a highly competitive field.
Addressing the Talent Gap
To address the shortage of skilled talent, organizations can invest in training and upskilling programs for existing employees. Offering specialized courses or certifications in data analytics can enable individuals to acquire the necessary skills and contribute to the organization’s data-driven initiatives.
Collaborating with universities and research institutions can also help bridge the talent gap by nurturing a pipeline of skilled professionals. Additionally, leveraging external consulting firms or partnering with data analytics service providers can provide access to specialized expertise when needed.
3. Data Privacy and Security
With increasing concerns around data privacy and security, organizations must ensure that sensitive information is protected throughout the data analytics process. The integration of large datasets and the sharing of information across departments or with external partners can introduce vulnerabilities if not handled securely. Data breaches not only result in financial losses but also damage a company’s reputation and erode customer trust.
Securing Data Assets
To address data privacy and security challenges, organizations must implement robust data protection measures. This includes employing secure data storage and transmission protocols, role-based access controls, and encryption techniques.
Regular security audits and employee training on data protection best practices are also essential. Organizations should also establish clear data governance policies that outline the rules and procedures for handling and sharing sensitive data.
4. Scalability and Infrastructure
As the volume and complexity of data continue to grow, organizations face challenges in scaling their data analytics capabilities.
Legacy systems and infrastructure may struggle to handle the massive amounts of data generated in real time. This can result in slow processing times, limited storage capacity, and inefficient data retrieval, hindering the organization’s ability to derive timely insights.
Building Scalable Data Infrastructure
To overcome scalability challenges, organizations can invest in cloud-based data storage and processing platforms. Cloud infrastructure offers the flexibility to scale resources up or down based on demand, eliminating the need for extensive hardware investments.
Implementing distributed data processing frameworks, such as Hadoop or Spark, can further enhance scalability and improve processing speeds. Regular evaluations of infrastructure performance and capacity planning are necessary to ensure smooth operations.
5. Integration of Disparate Data Sources
Organizations often face the challenge of integrating data from various sources, such as customer data, sales data, and social media data. Each source may have different structures and formats, making it difficult to consolidate and analyze the data effectively.
Without proper integration, organizations may miss out on valuable insights that could drive business growth.
Integrating Diverse Data Sources
To address this challenge, organizations can employ data integration tools that are capable of handling different data formats and structures. Applying data transformation techniques, such as data normalization or data standardization, can help align disparate datasets. Implementing a robust data management strategy that includes data governance protocols and data integration practices can ensure the seamless integration of diverse data sources.
6. Balancing Data Accessibility and Security
While ensuring data privacy and security is crucial, organizations must strike a balance between data accessibility and the need for enhanced security measures. Restricting access too heavily can impede collaboration and limit the usability of data insights. Conversely, lax security measures can expose sensitive information to unauthorized individuals, jeopardizing the organization’s operations.
Creating a Secure and Accessible Environment
Organizations should implement role-based access controls to ensure that only authorized personnel can access specific data. Data classification and labeling can help differentiate sensitive information from non-sensitive data, allowing for more granular access control. It is also essential to establish clear data access policies and provide training to employees on data handling best practices. Regular audits and monitoring of data access logs can help identify and address any potential security breaches.
7. Ethical Considerations and Bias
Data analytics can be influenced by inherent biases in the data collected or the algorithms used. Unintentional biases can lead to skewed insights and perpetuate discriminatory practices. Additionally, the ethical considerations surrounding the collection and use of personal data require careful attention to avoid violating privacy rights and legal regulations.
Addressing Ethical Concerns and Bias
Organizations should develop ethical guidelines and frameworks that govern the use of data analytics. Conducting regular ethical reviews of data analytics processes can help identify and rectify any potential biases or ethical concerns. Implementing diverse and inclusive teams that can challenge assumptions and biases can also mitigate the impact of inherent biases in data analytics.
Organizations must also comply with applicable data protection and privacy laws to maintain ethical standards.
Effective Strategies for Overcoming Data Analytics Challenges
1. Build a Strong Foundation
Invest in establishing a robust data infrastructure and data governance framework that ensures data quality, security, and accessibility. Lay the groundwork for data analytics success by implementing the right technologies and processes to support your analytics initiatives.
2. Invest in Talent
Acknowledge the importance of skilled professionals in the field of data analytics and invest in attracting, training, and retaining top talent. Upgrading the skills of existing employees and collaborating with academic institutions can help address the talent gap.
3. Embrace Agile Methods
Adopt agile methodologies to ensure the agility and flexibility needed to handle changing business requirements and evolving data analytics needs. Embrace iterative approaches that allow for quick feedback and adjustments as insights are derived.
4. Continuously Monitor and Improve
Regularly assess the effectiveness of your data analytics processes and tools. Monitor data quality, system performance, and the impact of data-driven insights on your business outcomes. Identify areas for improvement and implement enhancements accordingly.
5. Foster a Data-Driven Culture
Embed a data-driven mindset throughout your organization by promoting data literacy and creating a culture that encourages evidence-based decision-making. Empower your employees to leverage data analytics to drive innovation and efficiency.
Future of Data Analytics: Emerging Trends and Opportunities
With the rapid advancements in technology and increasing availability of data, the field of data analytics is constantly evolving. Here are three emerging trends that are shaping the future of data analytics:
1. Artificial Intelligence and Machine Learning
Artificial intelligence and machine learning techniques are revolutionizing the way data is analyzed and insights are derived. These technologies enable advanced predictive and prescriptive analytics, uncovering hidden patterns and making data analytics more accessible to a wider audience.
2. Data Democratization
Data democratization refers to the process of making data accessible to non-technical users throughout an organization. With user-friendly analytics tools and self-service platforms, individuals with little to no coding knowledge can explore and derive insights from data, democratizing the data analytics process.
3. Real-time Analytics
The ability to analyze data in real-time is becoming increasingly important in today’s fast-paced business environment. Real-time analytics allows organizations to make data-driven decisions immediately, responding to market changes and customer needs without delay.
As organizations continue to embrace data analytics, the challenges will evolve, but so will the strategies to overcome them. By addressing these challenges head-on and leveraging emerging trends, businesses can unlock the full potential of data analytics and gain a competitive advantage in a data-driven world.
Frequently Asked Questions
Data analytics can be a complex field with its own set of challenges. Here are some frequently asked questions about the challenges of data analytics:
1. How do you deal with data quality issues in data analytics?
Data quality is a common challenge in data analytics. To address this, it is essential to have proper data governance practices in place. This includes ensuring data accuracy, consistency, and completeness. Implementing data validation and cleansing processes can help identify and correct any errors or inconsistencies in the data. It is also important to establish data quality metrics and regularly monitor and measure data quality to maintain the integrity of the analytics results.
In addition, involving subject matter experts and data stewards in the data analytics process can help identify potential data quality issues and ensure data accuracy. By paying attention to data quality, organizations can improve the reliability and validity of their data analytics outcomes.
2. What are the main challenges in data analytics for small businesses?
Small businesses face unique challenges in data analytics due to limited resources and expertise. One of the key challenges is the availability of data. Small businesses may not have access to large amounts of data, which can affect the accuracy and reliability of the analytics results.
Additionally, small businesses often lack the resources to invest in advanced analytics tools and technologies, making it harder to analyze and interpret data effectively.
Another challenge is the skill gap. Small businesses may not have dedicated data analysts or data scientists on their teams, which can make it difficult to derive meaningful insights from data. Overcoming these challenges requires small businesses to prioritize data collection, invest in user-friendly analytics tools, and consider outsourcing analytics tasks to external experts if necessary.
3. How can organizations overcome the challenges of data privacy and security in data analytics?
Data privacy and security are critical considerations in data analytics. To overcome these challenges, organizations should implement strict data access controls and encryption measures to protect sensitive data. They should also comply with relevant data protection regulations and standards, such as the General Data Protection Regulation (GDPR).
In addition, organizations need to establish robust data governance frameworks that define data handling procedures, including data classification, anonymization techniques, and secure data storage. Regular security audits and vulnerability assessments can help identify and address any security loopholes. By prioritizing data privacy and security, organizations can gain the trust of their customers and stakeholders and mitigate the risks associated with data breaches.
4. What are the challenges of integrating data from different sources in data analytics?
Integrating data from various sources can be a complex task in data analytics. One challenge is the inconsistency in data formats and structures across different sources. Data may need to be transformed and standardized before it can be effectively analyzed. Another challenge is the availability of real-time data integration solutions, as some data sources may have delays in data updates.
To overcome these challenges, organizations can invest in data integration tools that offer data transformation capabilities. These tools can help automate the process of consolidating and aligning data from different sources. Establishing clear data integration strategies and ensuring data compatibility across systems can also streamline the integration process and improve the efficiency of data analytics workflows.
5. What are the challenges of scaling data analytics for large enterprises?
Scaling data analytics for large enterprises poses its own set of challenges. One of the main challenges is handling large volumes of data. Large enterprises generate massive amounts of data from multiple sources, making it challenging to manage and analyze. This requires implementing robust data storage and processing solutions that can handle big data.
Another challenge is the complexity of data analytics models and algorithms. Large enterprises may need sophisticated analytics techniques, such as machine learning and artificial intelligence, to derive valuable insights from their data. This requires skilled data scientists and advanced analytics tools.
Scaling data analytics also involves ensuring high-performance computing infrastructure, including powerful servers and data processing capabilities, to handle the computational demands of large-scale analytics tasks.
Data analytics can solve many problems, but it also comes with challenges. One challenge is the quality of data, which needs to be accurate and reliable. Another challenge is the complexity of analyzing data, as it requires specialized skills and tools. Additionally, data privacy and security are important concerns that need to be addressed.
However, despite these challenges, data analytics offers valuable insights that can drive business decisions and improve outcomes. By addressing the challenges and using data responsibly, organizations can unlock the full potential of data analytics and gain a competitive advantage. So, while there are hurdles to overcome, the benefits of data analytics make it worth the effort.