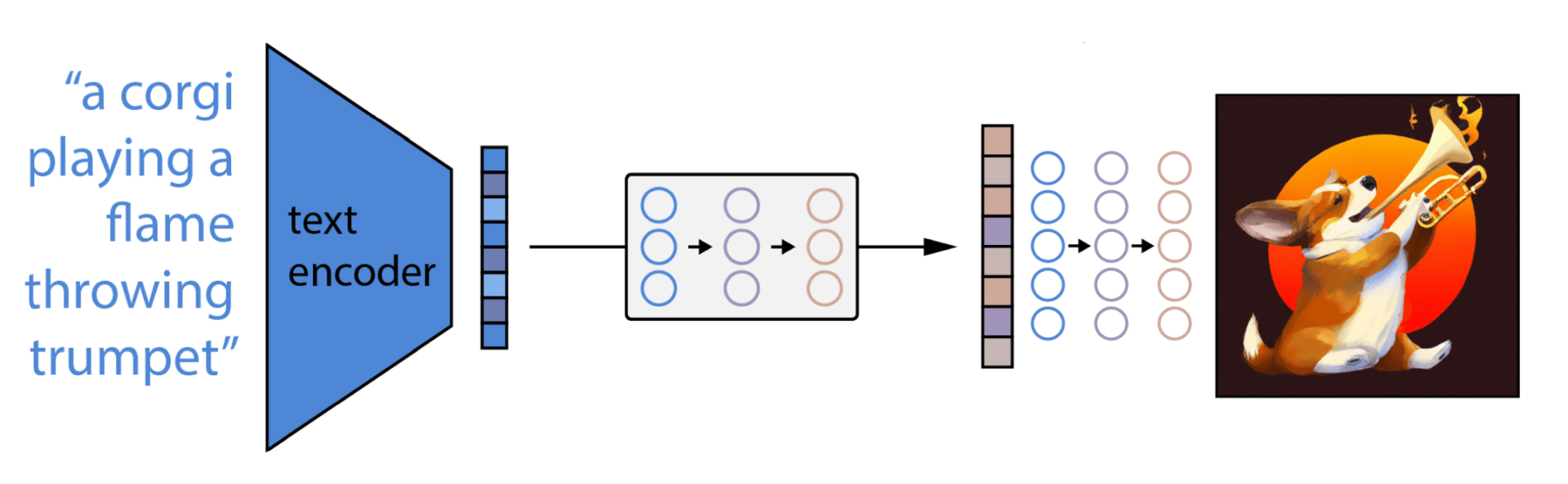
What’s The Training Process Like For DALLE2 AI? Welcome, young explorer, to the exciting world of DALLE2 AI! Have you ever wondered how a powerful AI model like DALLE2 is trained? Well, get ready to embark on a journey of knowledge as we delve into the fascinating training process of this cutting-edge technology.
First off, let’s start with the basics. DALLE2 AI is trained using a dataset that contains a vast amount of images and their corresponding text descriptions. The training process involves feeding this data to the AI model, allowing it to learn and understand the relationships between images and words.
During training, DALLE2 goes through countless iterations, analyzing and processing the images and text descriptions. It learns to generate realistic and coherent responses based on the given inputs. The more data it encounters, the better it becomes at understanding and generating accurate and contextually relevant outputs.
So, how does this training actually happen? Stay tuned as we explore the fascinating intricacies of DALLE2’s training process. Get ready to uncover the secrets behind how this exceptional AI model learns to create and imagine based on the vast world of images and descriptions it encounters. Let’s dive in!
In the training process for DALLE2 AI, several steps are involved to maximize performance. The first step is to prepare the dataset, ensuring it is diverse and representative. Next, pretraining the model is essential to learn general knowledge. Fine-tuning follows, where the model is trained on specific tasks. Finally, evaluation and validation are necessary to assess the model’s performance. By following this process, DALLE2 AI can achieve optimal results in various applications.
What’s The Training Process Like For DALLE2 AI?
Artificial Intelligence (AI) has rapidly advanced in recent years, and one of the most promising AI models is DALLE2. With its ability to generate high-quality images from textual descriptions, DALLE2 has gained significant attention in various industries. But how exactly does DALLE2 go from text to realistic images? In this article, we’ll dive into the training process of DALLE2 AI to understand the intricacies behind its impressive capabilities and explore the steps involved in training this cutting-edge AI model.
Understanding the Foundation: Pre-training
Before DALLE2 can generate stunning images, it goes through a process called pre-training. During pre-training, DALLE2 learns to predict the next word in a sentence by observing a vast amount of text from the internet. It leverages transformer-based architectures to understand the context and relationships between words, enabling it to generate coherent responses and generate realistic images later on. This initial training phase is crucial as it provides the foundation for DALLE2’s language understanding and image generation abilities.
During pre-training, DALLE2 also learns to extract relevant information from the text it receives. It grasps the nuances of language and develops a deep understanding of the diverse concepts conveyed through textual descriptions. Through exposure to a wide range of text, DALLE2 becomes proficient in understanding various topics, contexts, and styles, making it a versatile and robust AI model for generating images.
Pre-training allows DALLE2 to develop a rich vocabulary and grasp semantic relationships, ensuring it can accurately interpret and create images based on textual prompts. This foundational training process sets the stage for the subsequent steps in DALLE2’s training journey.
The Importance of Fine-Tuning
After the pre-training phase, DALLE2 undergoes fine-tuning, a crucial step in optimizing its performance. Fine-tuning involves training DALLE2 on specific datasets, enabling it to specialize in generating images based on particular domains or styles. This process ensures that DALLE2 becomes highly skilled in producing images that align with the desired characteristics specified in the training data.
During fine-tuning, a dataset of images is paired with corresponding textual descriptions. DALLE2 is trained to generate images that accurately represent the given text. By learning from this supervised training process, DALLE2 gains the ability to transform textual prompts into vivid, realistic images. Fine-tuning allows the AI model to enhance its visual understanding and precise image generation capabilities even further.
One of the key advantages of fine-tuning is its adaptability. By training on different datasets, DALLE2 can specialize in various domains and styles. For example, fine-tuning on a dataset of landscapes will enable DALLE2 to produce stunning nature-inspired images, while training on a dataset of portraits will equip DALLE2 to generate lifelike human faces. This flexibility makes DALLE2 a versatile tool for different industries and creative endeavors.
Gathering and Preparing Datasets
To ensure the effectiveness of DALLE2’s training, high-quality datasets play a vital role. The process of gathering and preparing these datasets requires attention to detail and careful curation. Datasets used in the training process should be diverse, representative, and of sufficient quantity to enable DALLE2 to learn and generalize effectively.
Textual prompts and their corresponding images form the core of the training datasets. These prompts are carefully chosen to cover a wide range of concepts and topics that DALLE2 should be able to understand and generate. The datasets can be created by human annotators, who provide the textual prompts and select or create the images that best represent the text. Alternatively, existing datasets available in various domains can be utilized, ensuring a diverse and comprehensive training experience for DALLE2.
Preparing the datasets for training involves preprocessing the text and images to ensure they are in a format that can be efficiently utilized by the AI model. This may involve cleaning the text, removing inconsistencies, and resizing or transforming the images. The quality of the datasets greatly influences the performance and capabilities of DALLE2, so meticulous attention to detail is essential in this stage of the training process.
Hyperparameter Optimization for Enhanced Performance
A crucial aspect of training DALLE2 lies in optimizing the hyperparameters, which are variables that govern the learning process of the AI model. These hyperparameters affect the speed, stability, and overall performance of DALLE2, and finding the optimal configuration can significantly enhance its capabilities.
The selection of hyperparameters involves experimentation and fine-tuning to strike a balance between underfitting, where the model fails to capture complex patterns, and overfitting, where the model becomes too specialized to the training data and loses generalization capabilities. Parameters such as learning rate, batch size, and model depth are adjusted to maximize DALLE2’s ability to generate high-quality images that accurately correspond to the given textual descriptions.
Hyperparameter optimization is an iterative process, involving multiple training runs with different configurations to identify the most effective combination. This process requires careful analysis and evaluation of the model’s performance metrics, such as image quality, diversity of outputs, and coherence with the given textual prompts. Through this iterative refinement, DALLE2 can achieve optimal performance and deliver impressive results.
Key Takeaways: What’s The Training Process Like For DALLE2 AI?
- The training process for DALLE2 AI involves feeding it with large amounts of data.
- During training, DALLE2 AI learns to generate images and text based on the input data.
- It goes through iterations of training to refine its understanding and improve its results.
- DALLE2 AI uses a technique called unsupervised learning, where it learns without explicit guidance.
- The training process requires powerful hardware and can take a significant amount of time.
Frequently Asked Questions
Are you curious about the training process for DALLE2 AI? Here are some common questions and answers to give you a better understanding.
How long does it take to train DALLE2 AI?
The training process for DALLE2 AI can vary depending on the size of the dataset and the computational resources available. Generally, it can take weeks or even months to train DALLE2 AI to achieve optimal performance. Training involves feeding the AI model with large amounts of data and allowing it to learn patterns and generate responses. This iterative process requires time and computational power to refine the model’s performance.
However, it’s important to note that the training time for DALLE2 AI is significantly faster compared to its predecessor, DALL-E. The improvements in algorithms and hardware infrastructure have accelerated the training process, making it more efficient.
What kind of data is used to train DALLE2 AI?
To train DALLE2 AI, a diverse range of data is required. This includes text, images, and other multimodal information. The training process involves exposing the AI model to a large dataset containing a variety of information, enabling it to learn and generate responses across different domains.
This data often comes from public sources, such as the internet, books, articles, and other text-based materials. Images are also utilized to provide visual context and enhance the model’s ability to generate relevant and coherent responses. By training on a diverse set of data, DALLE2 AI becomes more versatile in its understanding and generation capabilities.
How does DALLE2 AI learn during the training process?
DALLE2 AI learns through a process called unsupervised learning. In unsupervised learning, the AI model doesn’t require explicit labels or annotations to understand patterns in the data. Instead, it learns by automatically identifying and internalizing complex patterns and structures.
During the training process, DALLE2 AI uses a technique called self-attention, which allows it to focus on relevant parts of the data and understand the relationships between different elements. This enables the model to capture intricate details and generate coherent responses. Through multiple iterations of training, DALLE2 AI refines its understanding and becomes more skilled at generating contextually appropriate outputs.
Can DALLE2 AI be fine-tuned for specific tasks during training?
Yes, DALLE2 AI can be fine-tuned for specific tasks during the training process. Fine-tuning involves training the model on a smaller, task-specific dataset in addition to the initial general training. By providing the model with a narrower focus, it can learn to generate responses that are more optimized for a particular task.
For example, if the goal is to use DALLE2 AI for image generation, the model can be fine-tuned on a dataset specifically curated for image-related tasks. This helps the model generate more visually appealing and contextually relevant images. Fine-tuning provides the flexibility to tailor DALLE2 AI’s capabilities to meet specific needs in various domains.
What computational resources are required for training DALLE2 AI?
Training DALLE2 AI requires significant computational resources. The process involves running complex computations on powerful hardware, such as graphics processing units (GPUs) and tensor processing units (TPUs). These hardware accelerators are designed to handle the heavy computational workload and optimize the training process.
Additionally, ample storage capacity is necessary to store the large datasets used in training. Both the computational resources and storage capacity are critical for efficient and effective training of DALLE2 AI. Access to high-performance hardware and abundant storage is essential to maximize the model’s learning potential and achieve optimal training outcomes.
Summary
Training an AI like DALL·E2 is a complex process that involves two main stages. First, the model is trained on a massive dataset to learn patterns and generate images. Then, human reviewers provide feedback to fine-tune the model and ensure it aligns with human values. The training process is constantly evolving to improve the AI’s capabilities and ensure its responsible use.
Key Takeaways
Training the DALL·E2 AI involves a combination of data-driven learning and human review feedback. This allows the AI to generate high-quality images while preserving ethical guidelines. By constantly improving the training process, OpenAI aims to create a more capable and responsible AI system that benefits everyone.